Health Data Science Day 2025
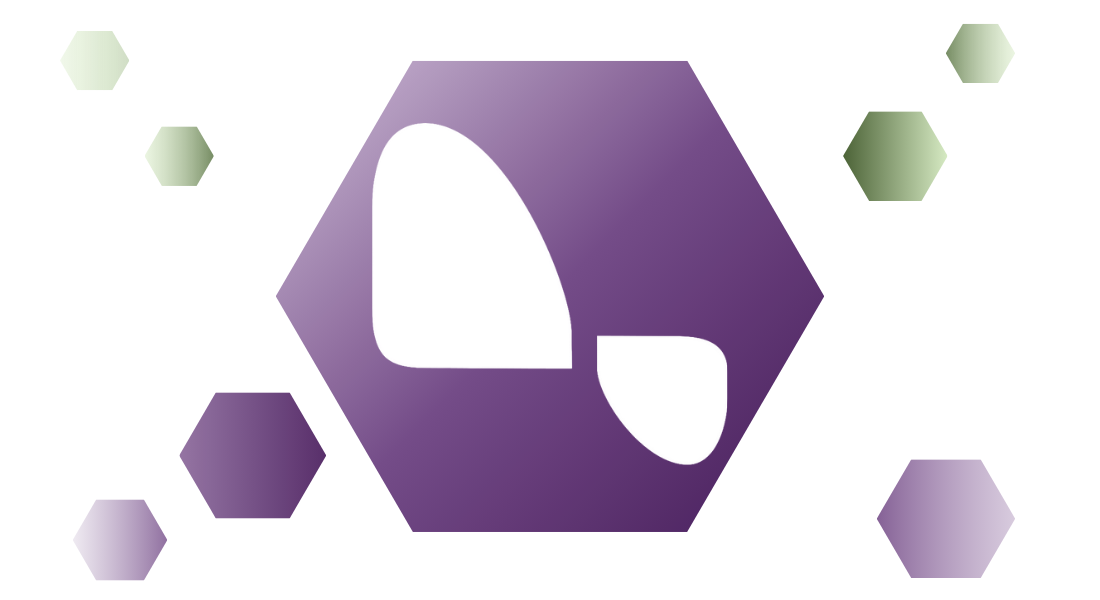
Health Data Science Day 2025 will take place on 6 November 2025 at the University of Copenhagen!
Health Data Science Day (HDSD) aims to bring together students, researchers, and industry employees that work across the wide field of health data science. The Center for Health Data Science (HeaDS) aims to assemble everyone from master's students, PhD fellows, early career researchers and established experts.
HDSD is a meeting place for those engaged or interested in health data science, no matter the path they took to get there.
Key information
Registration is free and open!
Speakers will be revealed on an ongoing basis - Keep your eyes on the HeaDS website, LinkedIn, and Mastodon to stay in the loop!
Important deadlines:
- Last day to register AND submit an abstract for a poster/lightning talk - 1 September
- Last day to register - 1 October
Health Data Science Day is a robust one-day conference:
Keynote Speakers – Two international keynote speakers will take the stage for 45 minutes each, with time built in for discussion.
Local Talent Talks – In an effort to highlight local(ish) talent, HDSD has invited 4 speakers across Denmark, Sweden, and Germany to give 15-minute presentations on their work.
Lightning talks – There are so many incredible researchers in and around Denmark. There will be a lightning talk showcase so we can hear from as many as we can. Talks will be 3 minutes each, challenging speakers to provide brief, informative, fun snapshots of their research. Attendees that submit poster abstracts will have the opportunity to opt in for lightning talk consideration. There will be a prize for the best lightning talk.
Poster sessions – There will be two reserved blocks of time for poster sessions. In each session, half of the poster authors will be stationed at their posters to talk to attendees and answer questions. There will be a prize for the best poster.
Networking – While attendees are encouraged to speak with their fellow conference-goers, we will host a reception following the second keynote speaker to allow attendees to connect with one another before going their separate ways.
Refreshments – HDSD will provide a light breakfast in the morning, coffee and tea throughout the day, lunch, an afternoon snack, and light fare during the networking session at the end of the day.
Keynote speakers:
Using Genetics and Omics for Prediction of Common Disease
Risk stratification is an important part of preventative management of common complex diseases such as cancers, heart disease and diabetes, with risk calculators based on lifestyle factors, blood biomarkers and family history. Common diseases all have an important polygenic etiology which means that the majority of people affected have no family history of the disease. More than 15 years ago, Naomi was the first to propose that results from genomewide association studies could be used to develop genetic risk predictors. Methods for improving genetic risk prediction as well as prediction from omics blood-based biomarkers continue to be a key focus of research for the Wray lab. The importance of such polygenic risk predictors should neither be oversold nor undersold. Naomi will review key advances in the methodologies and application of polygenic risk prediction and how risk prediction can be further enhanced by omics information.
Naomi Wray is the Michael Davys Professor of Neuroscience in the Department of Psychiatry, University of Oxford, UK and Professor of Quantitative Genetics at the Institute for Molecular Bioscience, University of Queensland, Australia. She is Director of the Pioneer Centre for SMARTbiomed (statistical and computational methods to advance research to transform biomedicine), a Denmark-Oxford collaboration, led from the University of Aarhus, Denmark. She is a Fellow of the Australian Academy of Science and Fellow of the Australian Academy of Health and Medical Sciences. She received the 2020 Ming Tsuang Lifetime Achievement Award from the international Society of Psychiatric Genetics. Her early training was in quantitative genetics applied to livestock. Her research interfaces development on new analytical methods and analysis of common complex diseases particular psychiatric disorders with a view to improved prevention, diagnosis, prognosis and treatments.
Unlocking the Potential of Health Data: Navigating the Challenges of Predictive Modeling in Clinical Decision-Making
The adoption of AI systems in medicine has become an essential asset for analyzing the vast amounts of multimodal health-related data generated daily. However, several challenges persist, including limited sample sizes, systematic biases, and the lack of interpretable and reliable predictions. To address these challenges, novel machine learning and bioinformatics algorithms are essential to support the analysis of the emerging complex multimodal biomedical data and their transition toward clinical applications.
Anne-Christin Hauschild heads the Institute for Predictive Deep Learning for Medicine and Healthcare at Justus-Liebig University Gießen. She was previously a junior professor at the Institute of Medical Informatics at the University Medical Center Göttingen and headed the Clinical Decision Support group. Her research is dedicated to developing machine learning methods and systems medicine approaches for analyzing biomedical data and their transfer into clinical practice.
Her group focuses on integrative analysis of multi-modal medical data, such as electronic patient records, molecular data such as gene and protein expression, and medical imaging data to support disease prediction and therapy optimization. ML technologies have been applied successfully in numerous health research domains, such as oncology, psychiatry, and cardiology. However, several challenges persist, impeding the translation of these advances into research and practice. Specifically, limited sample sizes, data privacy, and systematic biases within individual patient cohorts contribute to data scarcity and heterogeneity in medical registries and biomedical data. Additionally, the lack of interpretable and reliable predictions undermines trust in otherwise highly accurate models. Prof. Hauschilds group aims to address these challenges through employing and developing novel computational architectures and algorithms, including transfer learning to overcome data integration issues and accommodate small sample sizes, online and time-critical event prediction, federated learning for integrating distributed medical datasets and databases, as well as the use of explainable artificial intelligence methods to enhance model and prediction interpretability.
Local Talent Talks:
A Hub for Pharmaceutical Data Science Research and Education
This talk is an introduction to the people, organization, and ideas of the Center for Pharmaceutical Data Science Education (CPDSE). CPDSE is a close collaboration between the University of Copenhagen and the University of Southern Denmark. The goal of the center is to advance Danish education within pharmaceutical sciences through integration of data science. How can CPDSE help you? How can CPDSE work towards increased data literacy in the people and organizations across the pharma value chain? And what can we do with it?
Morten Lindow is the Head of Center for the Center for Pharmaceutical Data Science Education and Professor in the Department of Drug Design and Pharmacology. CPDSE is a close collaboration between the University of Copenhagen and the University of Southern Denmark. The goal of the center is to advance Danish education within pharmaceutical sciences through integration of data science.
Morten brings 17 years of experience to his work. He has turned numerous ideas into drug candidates, with five reaching clinical stages. A pioneer in computational design of oligonucleotide drugs, he has contributed to innovation through structure-activity relationships and kinetic models. Passionate about human-centered scientific collaboration, he has led projects at Roche and Santaris, integrating data science with drug discovery to advance RNA-therapy. His work has made significant strides in bioinformatics and organizational design, fostering cross-functional excellence.
Artificial Intelligence in Diabetes Research and Care
Adam Hulman is a Senior Researcher leading the Machine Learning & Clinical Prediction Lab at Steno Diabetes Center Aarhus, Aarhus University Hospital, Denmark. He is also an Associate Professor at the Department of Public Health at Aarhus University. He has a PhD and 15 years of experience in working with diabetes epidemiology. His team’s overarching goal is to turn health data into clinical insights and applications by using advanced statistical and machine learning methods. More specifically, the group works on the development and application of deep learning methods to be able to integrate clinical data of different types (tabular, images, time series, voice) in risk prediction of diabetic complications.
He is committed to hearing the users’ voices about AI and to building bridges between diabetes researchers, clinicians and the data science community. Adam is an active academic citizen: steering committee member of the European Diabetes Epidemiology Group, member of the EASD Guidelines Oversight Committee, and chairman of the Danish Data Science Academy’s Cross-academy Collaboration Committee. He is associate editor at Diabetolgia and PLOS Digital Health. He received the Danish Diabetes & Endocrine Academy Education & Networking Award for his outstanding engagement in diabetes research education and training of early-career researchers in 2024.
Human-centered Cyber-physical Systems: How can we build better intelligent systems?
Join Dr. Didem Gürdür Broo as she explores the evolution and challenges of cyber-physical systems in the Industry 5.0 era. This talk examines the critical intersection of connected, autonomous, and intelligent technologies, while questioning our relationship with data. Are we managing data effectively, or simply collecting it? Dr. Broo presents the five key dilemmas of data management—collection, sharing, trust, volume, and decision-making—and introduces three transformative mindsets (Design, Systems, and Future Thinking) that can help us build more human-centered intelligent systems. Discover how shifting our approach to technology development can create sustainable, effective solutions that prioritize human needs alongside technological capabilities.
Didem was at Stanford University as a Marie Skłodowska-Curie Fellow on Human-centered and Sustainable Cyber-physical Systems and at the University of Cambridge as a Research Associate in Cyber-physical Systems. Didem received a Ph.D. in Mechatronics from the Royal Institute of Technology.
Didem is an Institute of Electrical and Electronics Engineers Senior Member due to significant performance and excellence in science. Didem was also named as part of 100 Women in AI Ethics and Global Top 100 Innovators in Data & Analytics. Didem teaches at Oxford University and the University of Cambridge in a few courses and modules.
Didem cares about the future of the world and nature. Didem is a computer scientist who aims to make future cyber-physical systems interoperable and sustainable. An analytical thinker with a passion for design thinking, a researcher with a future perspective, an engineer who likes problems more than solutions, and a teacher who likes to play during lectures, Didem wants to bring new perspectives to designing the future intelligent machines.
Currently, Didem is an Associate senior lecturer and Assistant Professor at Uppsala University in the Department of Information Technology’s Division of Computer Systems.
Challenges and Chance of Mining Routine Care Electronic Health Records for Rare Diseases
Hospitals around the globe are gathering data in an increasing pace, yet we are starved for data, in particular when looking at rare diseases. How can that be, where are the roadblocks? The answer is data lack in data standardization, complex regulatory frameworks and also the particularity of rare diseases. In this talk, he will report on the experiences gathered from training machine models on routine care data for rare polyneuropathies and myopathies.
Richard Röttger is a Professor for Bioinformatics at the University of Southern Denmark (SDU) at the Department for Mathematics and Computer Science (IMADA) and head of the Computational Biology Group. He started at SDU in Summer 2014 as Assistant Professor, became Associate Professor in November 2017, and a Full Professor in November 2022. He has since been actively researching various kinds of machine learning of biomedical data.
He spent a 5 months on Sabbatical leave at the University of Cambridge at the Medical Research Council Laboratory of Molecular Biology (MRC-LMB) in the group of Prof. Madan Babu.
Before joining SDU, Richard received his PhD (Dr. rer. nat.) from the Max-Planck-Institute for Informatics, in Saarbrücken for my work on Active Transitivity Clustering. He was awarded an International Max Planck Research School (IMPRS) PhD fellowship for his research at the Max Planck Institute. Before my PhD studies, he studied computer science at the Technical University of Munich (TUM) and Technology Management at the Center for Digital Technology and Management (CDTM). During his studies he had two research visits at the University of California at Berkeley at the School of Information as well as at the International Computer Science Institute where his diploma thesis originated. His thesis on the completeness of gene regulatory networks was awarded with the prestigious Siemens prize.
Questions?
Please reach out to heads-admin@sund.ku.dk with any questions.